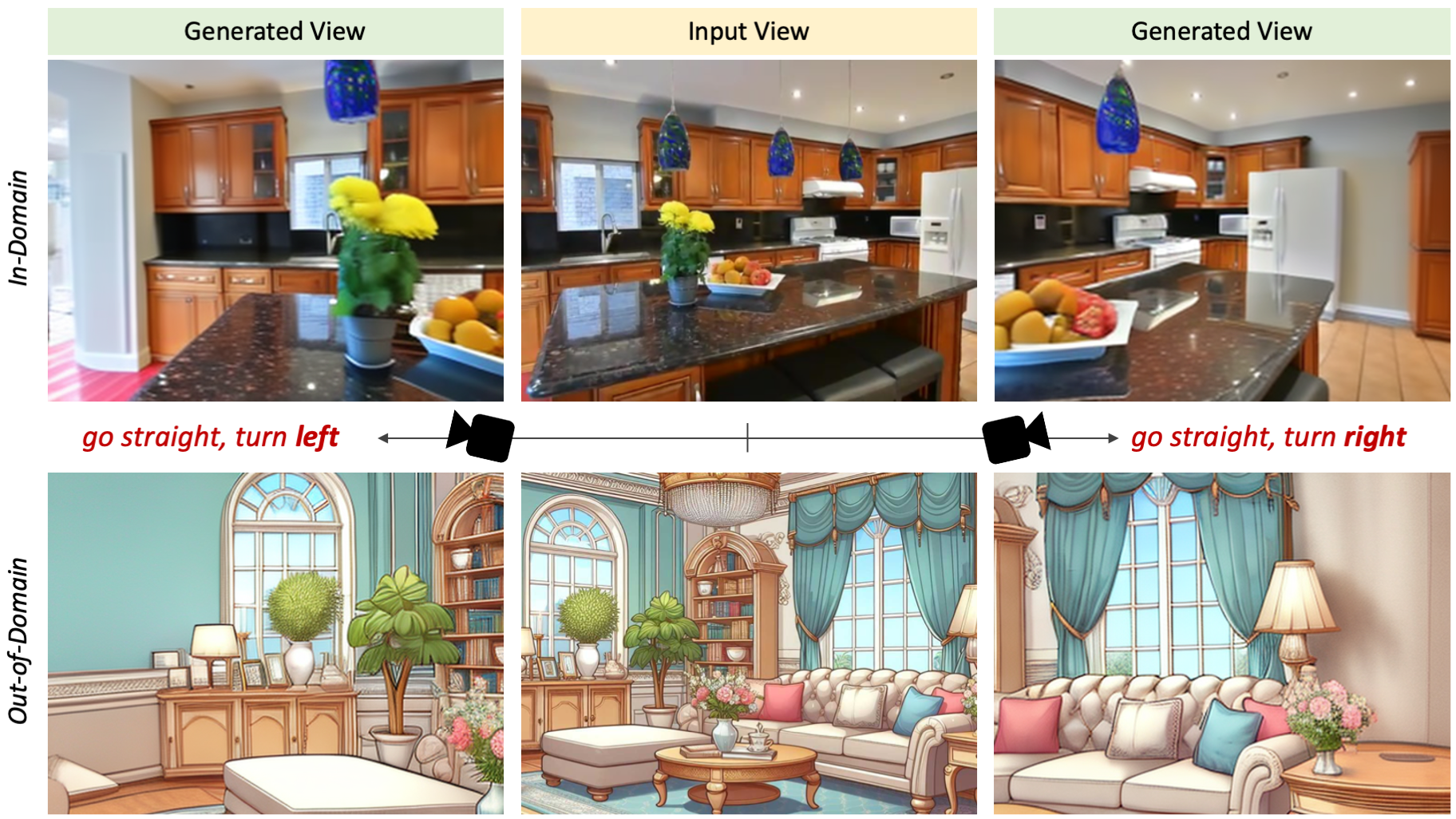
Application: Single Image to 3DGS
Our model can be applied to various downstream tasks. For example, given a single image, our model generates 3-4 novel view images, followed by feeding them into fast 3DGS reconstructors such as InstantSplat. Then we can easily obtain a 3DGS scene in 30 seconds.Warping-and-Inpainting vs. Ours
We introduce a novel approach where a diffusion model learns to implicitly conduct geometric warping conditioned on MDE depth-based correspondence, instead of warping the pixels or the features directly. We design the model to interactively compensate for the ill-warped regions during its generation process, thereby preventing artifacts typically caused by explicit warping.
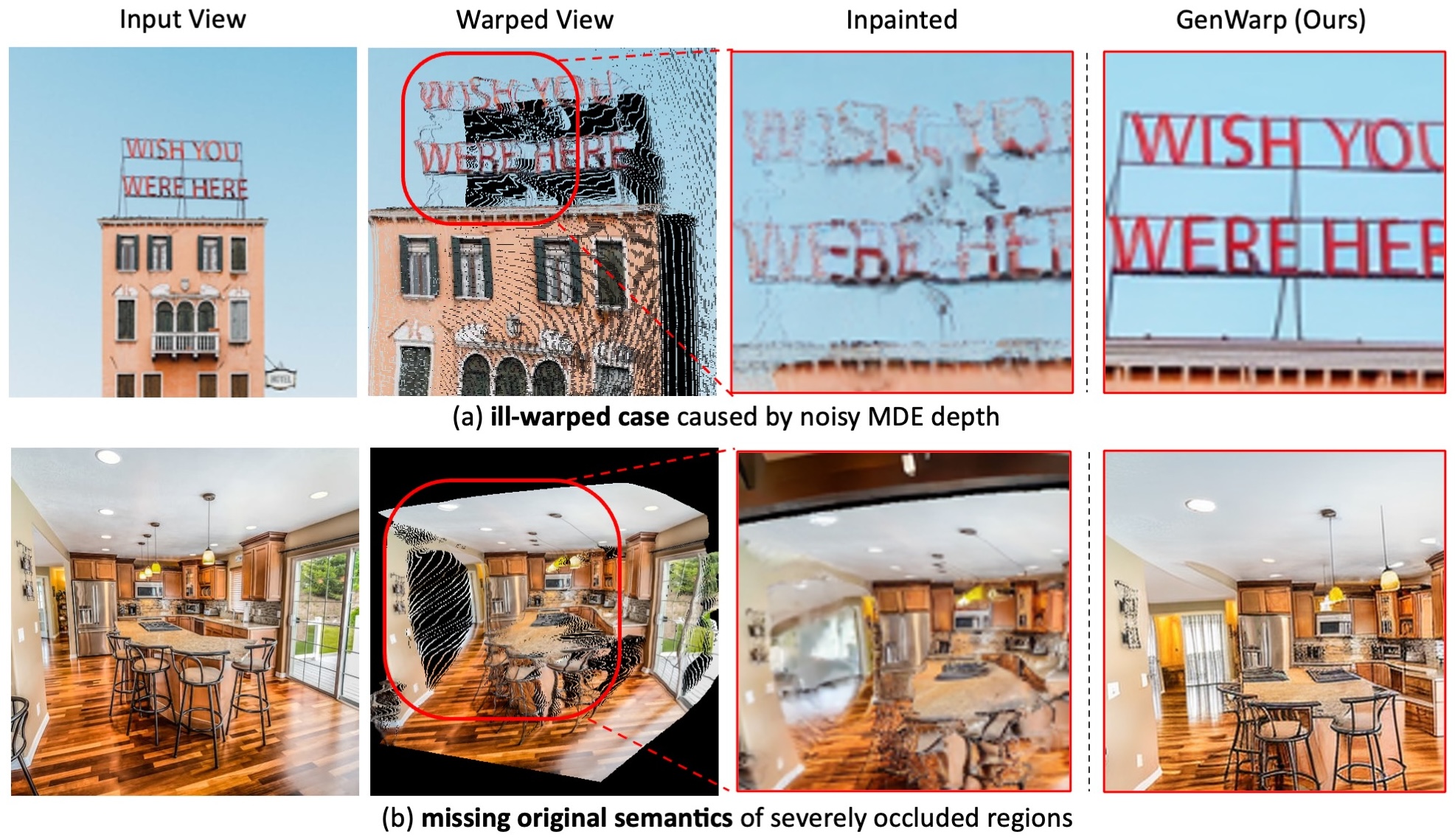
GenWarp Knows Where to Warp and Where to Refine
In our augmented self-attention, the original self-attention part is more attentive to regions requiring generative priors, such as occluded or ill-warped areas (top), while the cross-view attention part focuses on regions that can be reliably warped from the input view (bottom). By aggregating both attentions at once, the model naturally determines which regions to generate and which to warp.
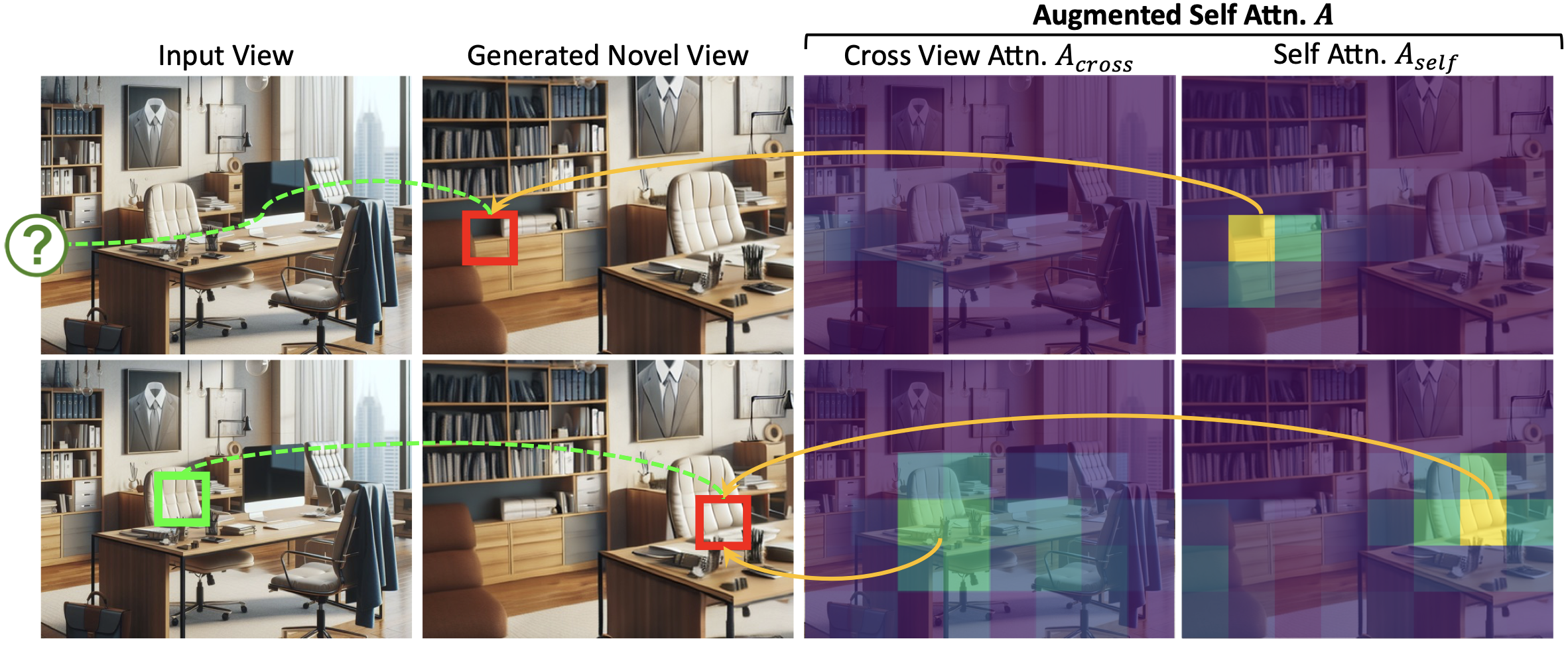
Qualitative Results on In-The-Wild Images
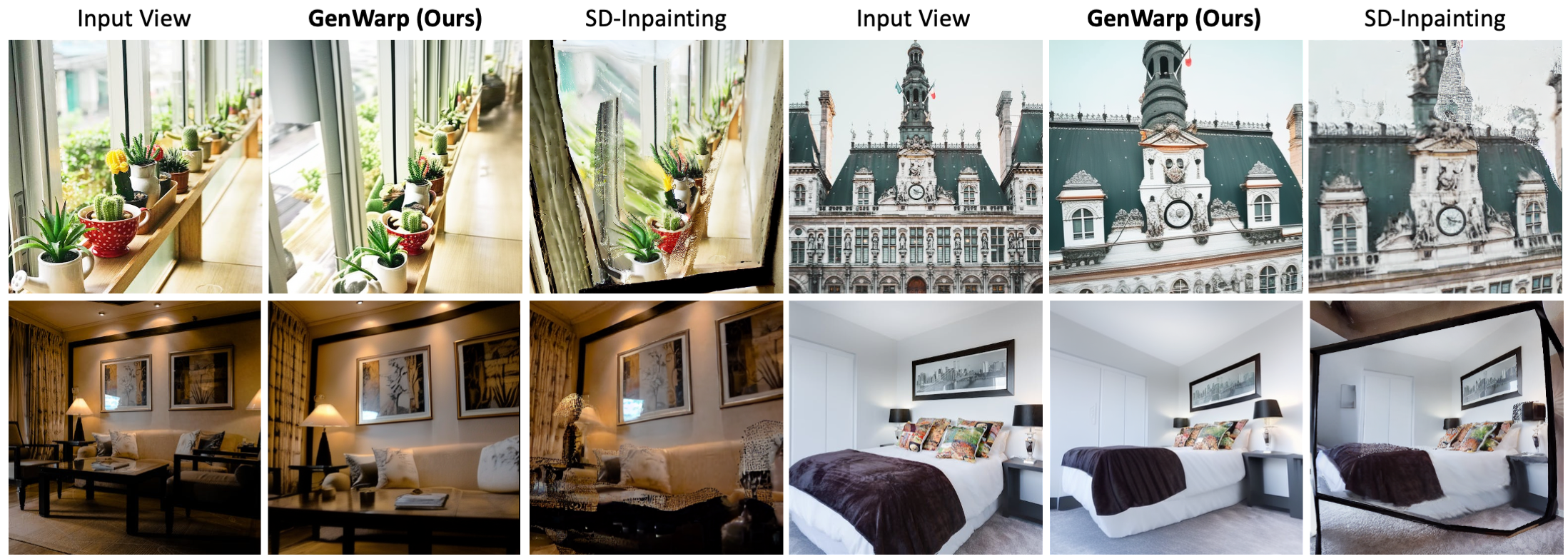
Overall Framework
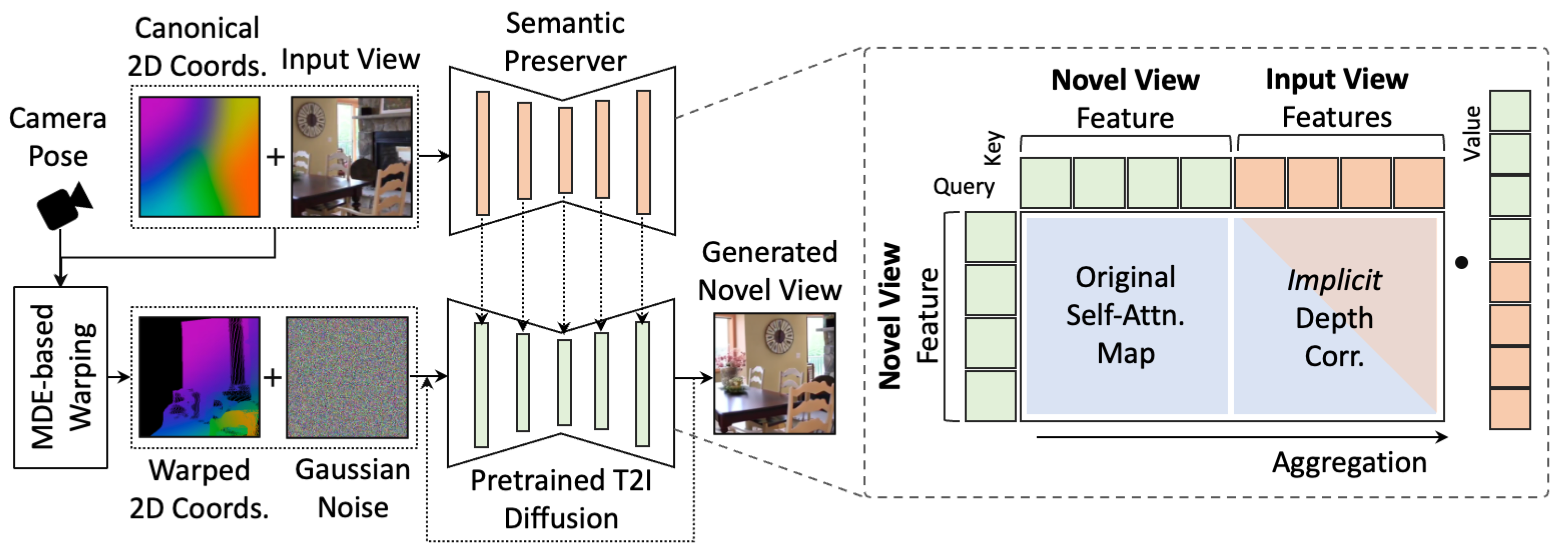
Abstract
Citation
Acknowledgements
We thank Jisang Han for helping with the 3DGS application in this project page. The website template was borrowed from Michaël Gharbi.